OpenAI's Whisper is another case study in Colonisation
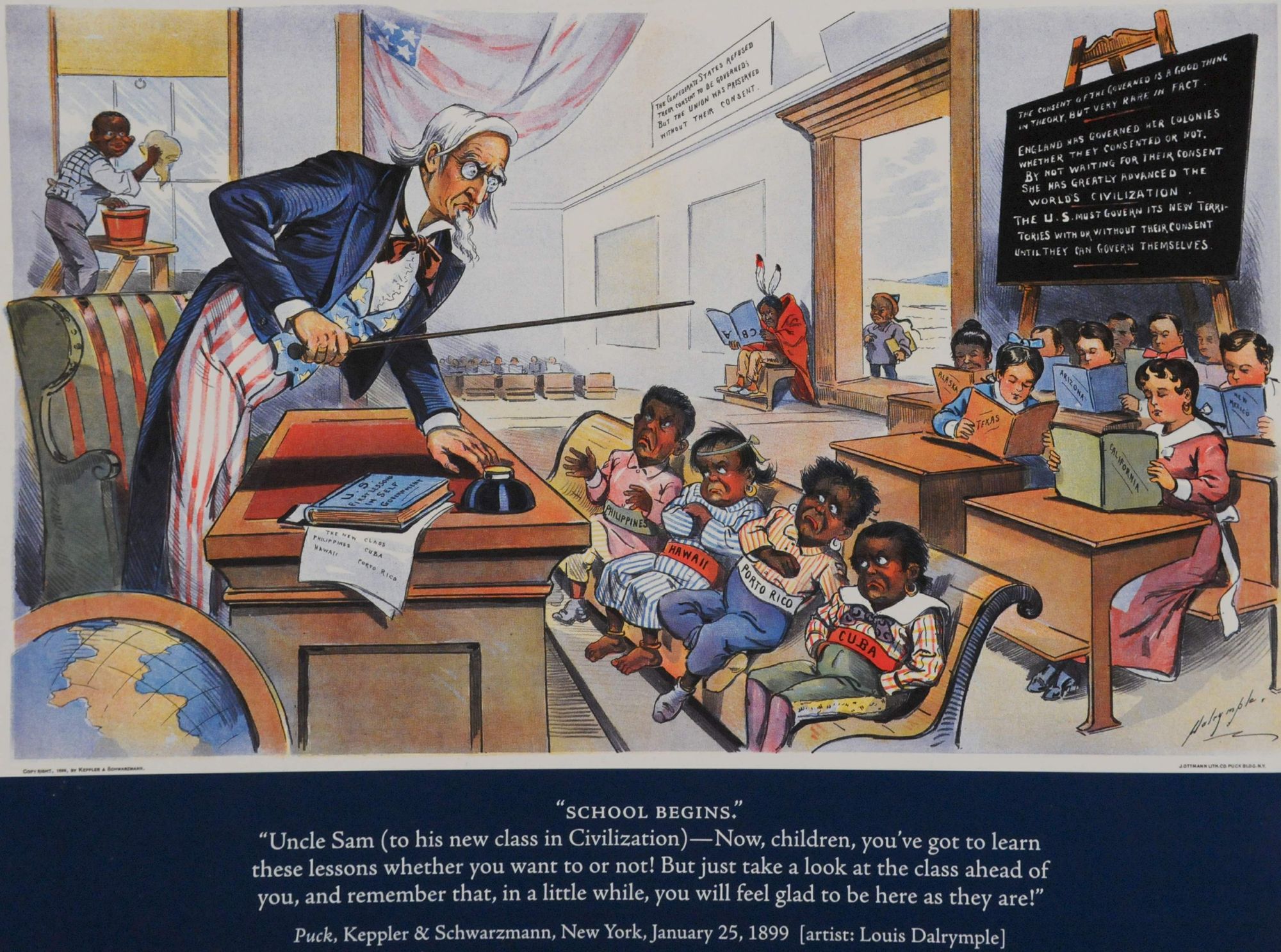
On 21 September OpenAI dropped Whisper, a speech recognition model trained on 680,000 hours of audio taken from the web. The highlight:
it enables transcription in multiple languages, as well as translation from those languages into English. We are open-sourcing models and inference code to serve as a foundation for building useful applications and for further research on robust speech processing.
The ability for a single model to transcribe in multiple languages is ground-breaking for natural language processing (NLP) technologies. With such bold statements, why didn't we hear more about Whisper from news outlets or social media? Twitter was instead dominated by critiques about Stable Diffusion and other generative art models that are infringing on copyright and appropriating the work of artists. Even big-name late-night hosts covered these models. John Oliver’s foray into generative AI ultimately led him to marry a cabbage and Trevor Noah’s more serious interview with OpenAI's CTO Mira Murati exposed the view that there were mostly positive outcomes from this technology with no critical discussion about the potential harm it could cause, making statements that images created from DALL-E were indeed art created not by a brush but by a digital tool.
In an interview with an engineering student who posted a model on Reddit that mimics the works of artist Hollie Mengert, we encounter a view that is shared too commonly in the tech industry,
I don’t really care if you think this is right or wrong. You either use Stable Diffusion and contribute to the destruction of the current industry or you don’t. People who think they can use [Stable Diffusion] but are the ‘good guys’ because of some funny imaginary line they’ve drawn are deceiving themselves. There is no functional difference.
Ultimately the engineering student says the technology is here and it is inevitable. These fatalistic views and approaches need to be rejected. We recognize that AI is important and here to stay and an important tool for society, but we must reject the notion that it is impossible to do this in a way that is respectful, that doesn't infringe on copyright, does not appropriate cultures, and that doesn't privilege the haves over the have nots.
The appropriately named Whisper model, however, was just that, a whisper unheard amongst the hype of generative AI and massively large language models aiming to transform our future.
New Zealand's approach to AI
A small, non-profit Māori organisation in Aotearoa New Zealand heard the Whisper and were intrigued. Te Reo Irirangi o Te Hiku o Te Ika, aka Te Hiku Media, have spent the last 32 years working to help revitalise and promote te reo Māori, the language of the indigenous Māori people of Aotearoa. Today, Te Hiku Media are building NLP tools such as speech recognition to accelerate the revitalisation of te reo Māori.
The Māori language has suffered over a century of harm. It was once forbidden to speak te reo Māori. Many Māori living today have grandparents who were beaten in school for speaking their native language.
One challenge in building NLP tools for indigenous languages is the need to code-switch between the indigenous language and the language of their colonisers. For te reo Māori, English words, phrases, and sounds commonly enter daily discourse in te reo Māori; likewise, Māori place names are prevalent in NZ English discourse, just try asking Siri to take you to ‘Whangamatā’. Furthermore, New Zealanders are using more and more Māori words and phrases in daily conversation, in flight safety videos, and on chocolate wrappers. The ability to transcribe in multiple languages was more than a whisper to our ears, it was a call to action to assess these bold claims and explore the ramifications of open-sourcing such a profound technology, especially for indigenous languages.
The Māori language has suffered over a century of harm. It was once forbidden to speak te reo Māori. Many Māori living today have grandparents who were beaten in school for speaking their native language. Despite a growing appetite to learn te reo Māori, New Zealand is not short of racists demanding people "Speak English!" or boycotting companies like Whitakers for using te reo Māori on their packaging. The oppression of indigenous languages is why we have concerns about non-indigenous groups building tools like Whisper.
A few of our data scientists tried Whisper on te reo Māori videos from YouTube. Their initial reaction was, "Wow it works!" A more critical assessment of Whisper by our Māori data experts saw that it sort of worked but it was terrible. Still, this is concerning, that a non-Māori organisation thought it was okay to create a Māori speech recognition model and open it to the public.
Concerns around Data
Our team studied the Whisper paper because it could potentially be a game changer (the thing you say every other month when Big Tech drops another "AI" model). We got excited about the opportunity to fine-tune Whisper to train a bilingual Māori and English model. But the more concerning question, raised by Whisper's ability to transcribe te reo Māori, was where did Whisper get its data? The Whisper model was trained with 1381 hours of te reo Māori and 338 hours of ʻōlelo Hawaiʻi. The paper doesn't explicitly state where this data was taken from, but one can infer the data was scraped from the web. Scraping data from the web is particularly alarming when they don't have the right to use that data or to create derived works from it. Of course if you upload videos to certain platforms like YouTube, you,
grant to YouTube a worldwide, non-exclusive, royalty-free, sublicensable and transferable license to use that Content (including to reproduce, distribute, prepare derivative works, display and perform it).
Most, if not all, “free” services offered by Big Tech require you to give them exclusive rights to create derived works from your data, derived works such as models like Whisper and GPT-3.
The Whisper model was trained with 1381 hours of te reo Māori and 338 hours of ʻōlelo Hawaiʻi. The paper doesn't explicitly state where this data was taken from, but one can infer the data was scraped from the web.
We've reached out to a few people with connections to OpenAI and we haven't heard back. An important dataset in Whisper is the multilingual-speech data set FLEURS, which contains 2009 sentences translated into different languages and read by people. The paper is specifically about the data set, but it fails to disclose how exactly they got the data. We emailed one of the authors of FLEURS but have not heard back. Transparency should be required when it comes to the origins of data used in training models, especially models that are placed in the public domain.
In 2018, Lionbridge were soliciting people to read phrases in indigenous languages. Emails were sent to colleagues and entire language departments at universities in Hawaiʻi, Sāmoa, and Aotearoa to read phrases for$45-$90 USD/hour. We now believe it was Google behind the campaign because we were recently informed that Google’s Cloud Platform has some third party arrangements with Lionbridge. The FLEURS paper is possibly this data set, which has Google and META listed as co-authors. This is extractive capitalism. The rhetoric found in these emails coming from Lionbridge and recruiters who had no vested interest in our indigenous languages was laughable,
"We strongly believe in preserving a large diversity in languages and cultures and so we would kindly ask your support in keeping the Hawaiian language alive."
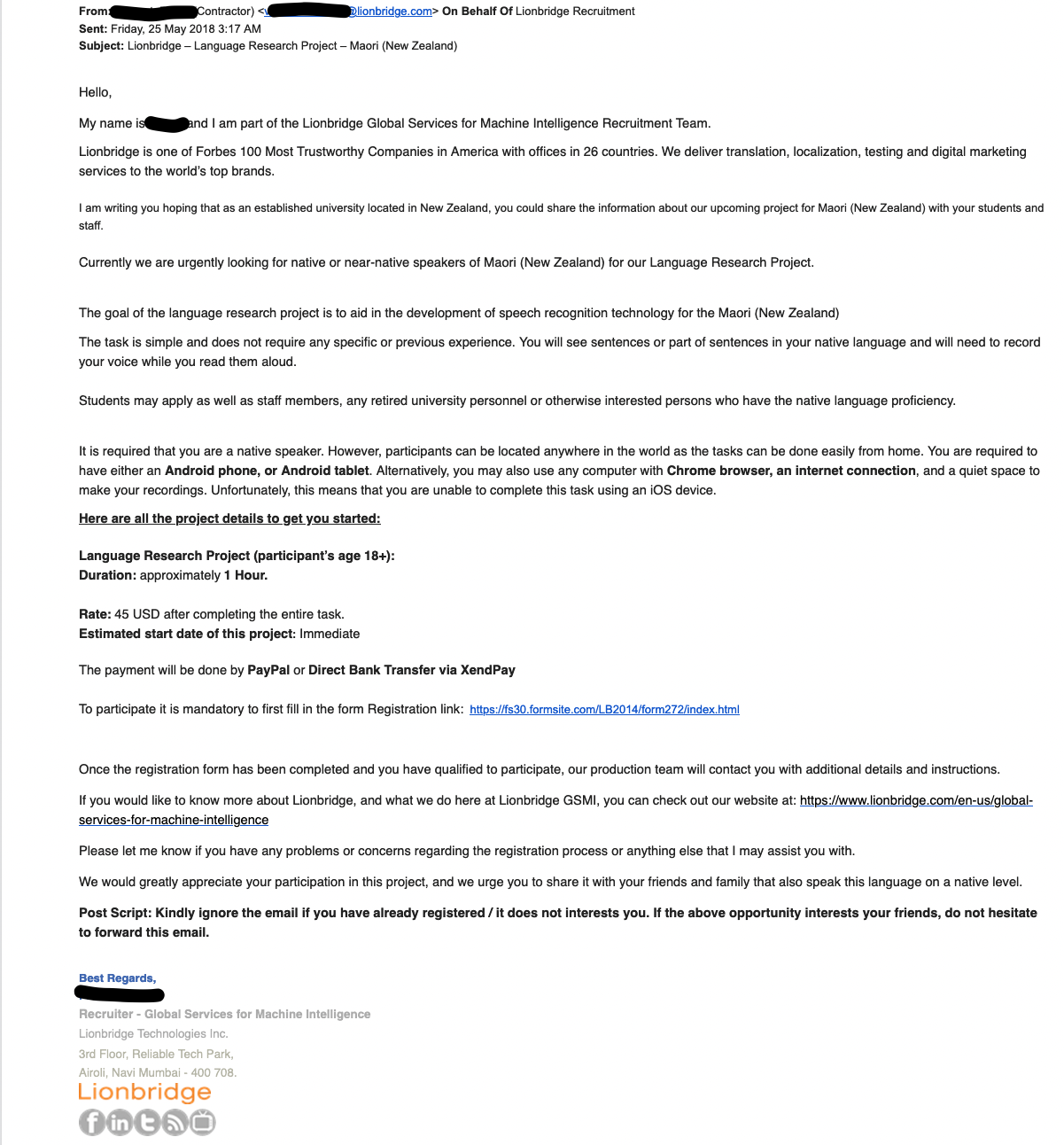
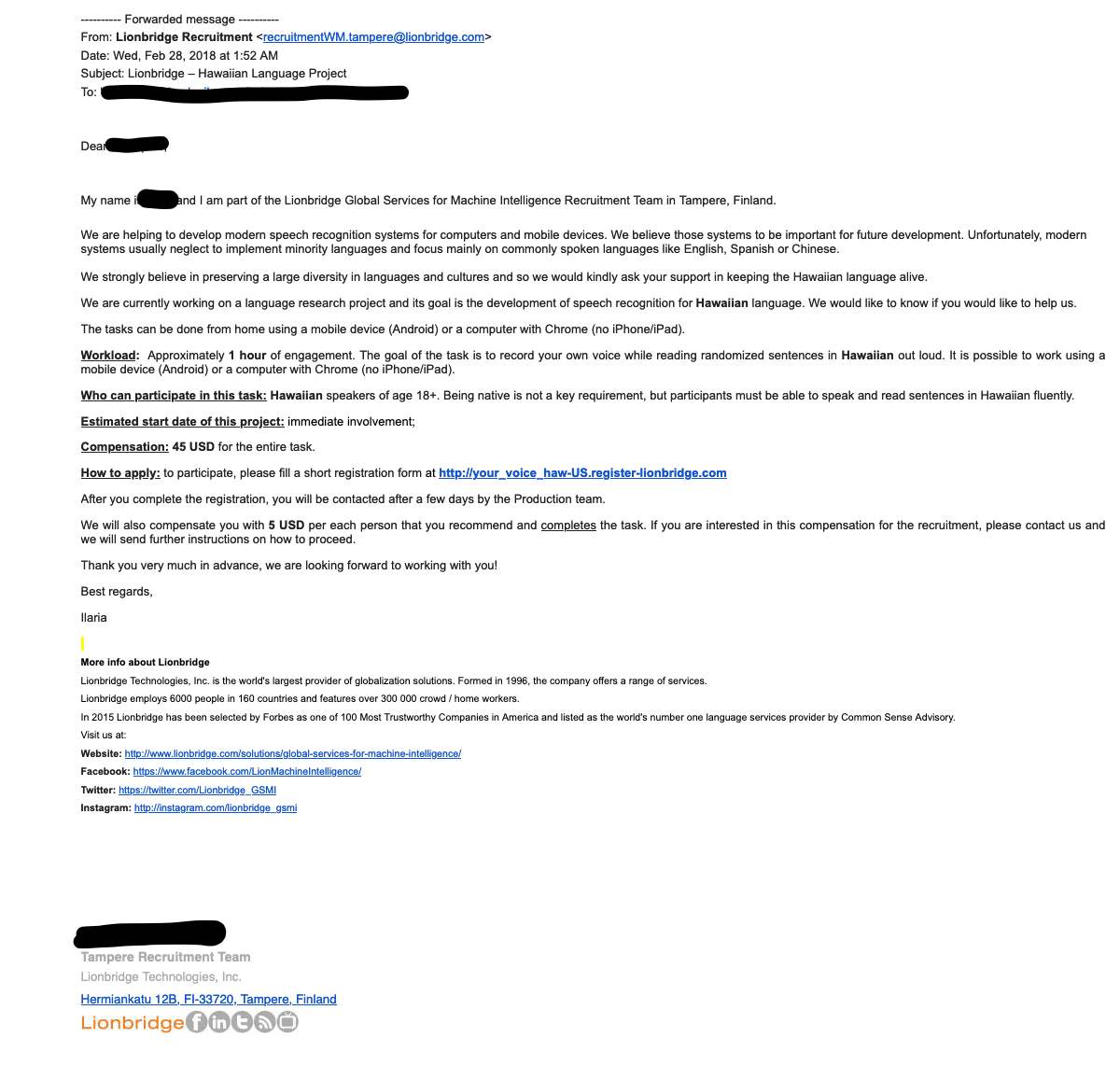
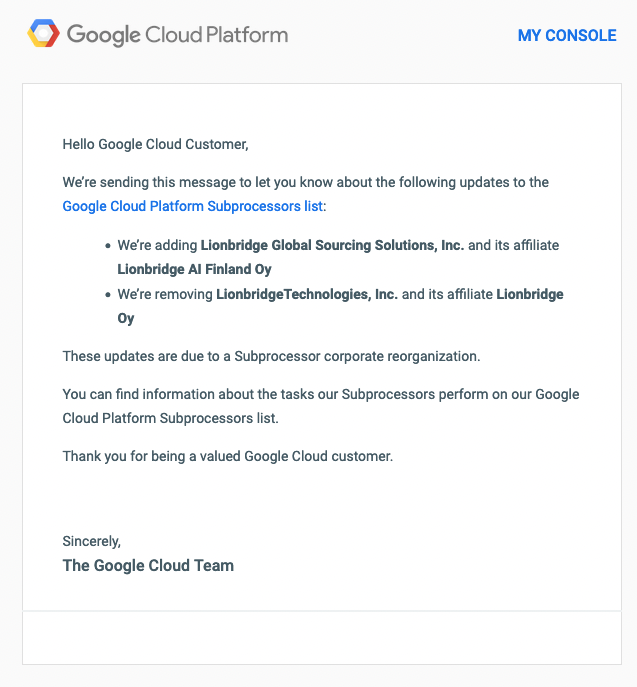
The main questions we ask when we see papers like FLEURS and Whisper are: where did they get their indigenous data from, who gave them access to it, and who gave them the right to create a derived work from that data and then open source the derivation? The history of colonisation and how actions like this do more harm than good is clear: Indigenous Data Sovereignty in the Era of Big Data and Open Data, Big Data May Not Know Your Name. But It Knows Everything Else, Indigenous Data Sovereignty. For our organisation, the way in which Whisper was created goes against everything we stand for. It's an unethical approach to data extraction and it disregards the harm that can be done by open sourcing multilingual models like these. It is problematic to only focus on the "good" that AI has to offer. Why would our organisation use something like Whisper, or could we ever use it in a way that doesn’t go against our values?
Damned if you do, damned if you don't
Having natural language processing tools for our languages is critically important to their survival in a digital society, and Aotearoa agrees, which is why the Papa Reo project was supported under the Strategic Science Investment Fund. But when someone who doesn't have a stake in the language attempts to provide language services, they often do more harm than good. Take Google Translate for example. Many New Zealanders have bastardised te reo Māori by asking Google to translate their English into Māori, including a mayoral candidate. When is a technology safe for public use? Who quality assures the Māori language translator at Google and decides that it works sufficiently enough to not generate poor quality te reo? We need to ensure we don't further harm te reo Māori with new technologies. That's why at Te Hiku Media we don’t release any product, service, or model that hasn't been vetted and quality assured by our Māori language and data experts. If we release a synthetic voice that mispronounces te reo Māori, we further damage the language already negatively influenced by English vowels and intonation.
The pace of AI research is depressingly fast. Depressing because currently the Ultra Wealthy are the ones pioneering the research, in some cases backed by the Effective Altruism movement. It's as if our only strategy is to sit patiently and wait to have another model shared with us from Big Tech and then spend entire conferences and research careers probing and prodding models trained by our Tech Lords.
If we really are concerned about Whisper, then we should evaluate it and see what harm or good it could do. In October, just a couple weeks after Whisper was published, our entire team spent about 6 weeks building a bilingual te reo Māori and NZ English model by fine-tuning Whisper.
Our first job was to quantify just how poorly Whisper off the shelf transcribed te reo Māori speech because qualitatively we knew it was terrible. We measure word error rates (WER) using a "golden dataset," a hand-curated corpora of te reo Māori speech that we classify as essential for any te reo Māori automatic speech recognition (ASR). The dataset includes native speakers, many of whom have passed, as well as highly proficient second language learners. Our current ASR, built using Mozilla’s DeepSpeech, performs with a 53% WER on the golden dataset, while the large Whisper model set to Maori [macron intentionally left out because Whisper does] language mode performs with a 73% WER. Whisper performs poorly on te reo Māori as expected. Below are some noteworthy examples comparing our current ASR and Whisper Large. These examples come from Te Reo Irirangi o Te Hiku o Te Ika's Legacy Collection of interviews recorded for radio decades ago.
current | whisper |
kāore rawa atu | Kauneroa ati! |
Whisper fails to transcribe correctly, but its transcription is acoustically similar to the target sentence.
current | whisper |
kia ora | ស្ ស្ ស្ ស្ ស្ ស្ ស្ ស្ ស្ ស្. |
Whisper spits out characters not part of the Māori language. This could be attributed to the poor audio quality of the utterance.
There were some edge cases in our initial experiment where Whisper performed better on the golden data set than the existing ASR.
current | whisper |
tēnā | Oh yeah, yeah, nah. |
This example demonstrates how Whisper can transcribe English while our existing ASR does not.
current | whisper |
nā te mea he tata rā te pōti e kōrero tēnā ko tetahi iwi | Nā te mea, te social anthropology he kōrero tēnā o tētahi iwi |
Whisper was able to transcribe te reo Māori and English in this utterance where the speaker code switches between the two languages.
Our initial investigation into Whisper got us excited about the opportunities. It also raised many concerns. Who else is fine tuning Whisper for an indigenous language? Are they indigenous language communities, or are they outsiders looking to capitalise on indigenous languages? Our team discussed and debated at length the ethical implications of using a model like Whisper. Te Hiku Media’s position is absolutely clear we would never use a model like this in production because it goes against our Kaitiakitanga License and it goes against all that we stand for. As far as we are aware there were no Māori or Hawaiians involved in making this model, and indigenous data were scraped from the web and used to create this model. We assume that OpenAI and the researchers had no right to use indigenous data in this way. If this assumption is incorrect, then who gave them that right and did they have the authority to give that right?
Our organisation has built deep trust in our community over the past 32 years. If we used a model like Whisper, or make similarly poor decisions, we can lose that trust in seconds. But could we be left behind if we don't embrace these types of technologies created from extracting data from the web? Will someone else, most likely a non-Māori group, use Whisper for te reo Māori if we don't?
Whisper isn’t a blood diamond. It doesn’t have lithium batteries like our mobile phones or the laptops our team use for their work. But it was trained on hardware with minerals extracted in ways that harm the environment and commit human rights violations. We use fossil fuels stolen from African nations and we eat meat grown on paddocks that were once native forests. How far removed from the problem does one need to be so that the ethical lines can no longer be visible?
Valuing and Respecting Data
Our quick evaluation of Whisper, and the existential threat it could impose, justified our decision to fine-tune Whisper using the data we look after under our Kaitiakitanga License. We treat data as taonga, something to be respected and revered. One could argue that data is like a family member, and it is your whakapapa (genealogy). We respect data in that we look after it rather than claim ownership over it. This is similar to how indigenous peoples look after land. We only use data in ways that align with our core values as an indigenous organsation and in ways that benefit the communities from which the data was gathered.
We respect data in that we look after it rather than claim ownership over it. This is similar to how indigenous peoples look after land.
Rather than extracting data at scale, training models, and then asking the research community to find the bias in them, we've taken the approach of having a deep and holistic understanding of the data in our corpora. Any one individual in our team doesn't know everything about the whakapapa of our data, but collectively we have full coverage. We know where the data comes from, when it comes from, who the speakers are, who they're related to, how the data was processed, how it was collected, and where it is stored. When we see errors in our models we can often pin point the causes and sources with ease. We think our approach to data is what has enabled us to accomplish what we have to date, and we hope the rest of the industry will at least learn from our ways of respecting data.
With our approach to data, we set out to fine tune Whisper to see if we could build a practical bilingual Māori and English ASR. Practical, in our experience, is a model that performs with a lower than 15% WER. We've found that a WER below 15% is good enough to use an ASR to assist people with transcribing audio. But 15% is relative and highly context-dependent. As mentioned, our ASR performs with a 53% WER on our golden dataset. In training, however, we report a ~15% mean WER on a holdout dataset. That training value is a good indicator for us. Furthermore, the fact that we qualitatively know our ASR works well for a number of contexts, we can base our qualitative judgment off a 53% WER on the golden dataset.
Our team set out to label as much bilingual speech as possible using a tool we built called Kaituhi. Our labelled te reo Māori speech corpus is upwards of 500 hours, but we decided to fine tune Whisper on about 125 hours of labelled speech including te reo Māori, NZ English, and bilingual speech. Our fine-tuned large Whisper model achieves a 38% WER on our golden dataset. Below are some examples.
current | fine-tuned whisper |
he aha te take nā te take | He aha te take? He aha te take? |
Not only did the fine-tuned model perform better, it also provided punctuation.
current | fine-tuned whisper |
e ngā tōmuri haere mātou kia kite haere i ērā nā ko tō | Āe, āe, kua mauria haere mātou kia kite haere i ērā mea katoa. |
The fine-tuned model could even transcribe and punctuate native speaker utterances that are less intelligible to the untrained ear.
current | fine-tuned whisper |
ko mea mai a tai ki a ko te mārama rātou | Hō, ko mea mai ētahi ki a au, "Whuu, te mārama rā tōku!" |
Even when the current model had a lower WER than the fine-tuned model, the latter provided a more accurate verbatim transcription.
Our golden dataset doesn't have much bilingual speech, so we also prepared data to measure against. To report on the performance of our bilingual model, we segmented our data into different contexts. We decided to break speech into four categories: Māori native speakers, Māori contemporary, bilingual, and NZ English. Native speakers are valued in indigenous communities especially where there are few native speakers left. Ultimately our goal is to bring back the native sound and language that was lost through colonisation, so it's important that native speakers are a key part of our corpora and how we evaluate NLP tools for te reo Māori. For practical uses of te reo Māori today, we need to measure against contemporary te reo Māori as well as NZ English and of course scenarios where the two are interchanged.
In the above chart, the performance of our existing DeepSpeech-based model is compared with various Whisper models. The Whisper model performs worse overall out of the box, but if we fine-tune Whisper using our data its performance drastically improves. Using our carefully curated training data the Whisper model is considerably better, even on te reo Māori, than our existing DeepSpeech-based model.
A Cautionary Tale
It seemed too easy: take an existing model from Big Tech, fine-tune it on some data, and all of a sudden you've got a powerful piece of technology that can transform an entire industry. Maybe it wasn't that easy. We've got an great team of data scientists, engineers, data specialists, and Māori language experts. We have the hardware, 4 x 80GB A100s connected via a DGX board with a 128 core CPU, to run Whisper and fine tune it. We have thousands of hours of audio data, some labelled and most unlabelled, and we have a team who have intimate knowledge of the data and know where to direct their energies to label data required to achieve this outcome in a short amount of time.
Many indigenous and marginalised communities don't have the resources or ability to benefit from open source because they are continually oppressed by systemic racism, governments, colonialism, and capitalism.
One could say we are privileged, except we’ve worked extremely hard for decades being innovative while being underfunded and under-resourced. But indigenous and marginalised communities often don't have privilege, and that's why open sourcing models like Whisper is concerning. In theory the open source movement aims to democratise technology like machine learning, which ultimately should be making tech more accessible and usable for minority groups. In practice, only those who have internet, a computer, the education, and other resources ubiquitous among Western countries and certain demographics are able to take advantage of the open source movement. Many indigenous and marginalised communities don't have these resources or ability to benefit from open source because they are continually oppressed by systemic racism, governments, colonialism, and capitalism. The open sourcing of large language models and Whisper best serves those who already have a foot in the industry, which is mostly White and Asian men. Just look at the demographics of NeurIPS 2022, "We observe that men (he/his pronouns) represent more than 75% of the proposed speakers. White and Asian speakers represent more than 90% of the speakers."
While individuals within Big Tech might genuinely believe they're supporting minority languages, the reality is that their actions create more opportunities for non-indigenous peoples and corporations than they do for the minority communities themselves.
Authoritarian governments, for example, have the power to use models like Whisper to improve their existing technologies, some of which are used for surveilling indigenous groups. They have the means to take a model like Whisper, fine tune it, and build tools to enhance their surveilling. We note that in a recent update to Whisper, the authors mention issues of surveillance, but they "expect" the existence of other limitations might prevent misuse of Whisper.
Large corporations also have the resources necessary to take these types of models and create products and services with them. Amazon recently created a Polly voice called Aria that can say some words in te reo Māori. The same audio produced using Aria clearly shows Amazon is hoping to provide a service to call centers and other places where speech synthesis is important. Big Tech clearly have an interest in providing te reo Māori as a service because it would open large economic opportunities to win many government and education contracts. Issues of Data Sovereignty might prevent this sort of extractive capitalism, but Big Tech are hoping to work around them.
Amazon, Google, and Microsoft are all building data centers in Aotearoa. These companies assume that by simply building a data center in Aotearoa they will meet Māori and New Zealand data sovereignty requirements, but that's not the case. While the US initially lost its appeal to extradite emails from Microsoft’s Ireland data centers, the new CLOUD Act,
allow[s] federal law enforcement to compel U.S.-based technology companies via warrant or subpoena to provide requested data stored on servers regardless of whether the data are stored in the U.S. or on foreign soil.
If your data is stored under the services of an American corporation, the US government can ultimately get access to your data. Furthermore most for-profit companies are accountable only to their shareholders, and their ultimate purpose is to return wealth to shareholders. They are not accountable to indigenous communities, and that lack of accountability is another way in which Big Tech will never align with data sovereignty.
If anyone is to profit from te reo Māori it should be Māori and Māori alone, especially considering the fact that non-Māori once sought to make the Māori language extinct.
There is a growing te reo Māori economy as the language becomes more ubiquitous and as the government aims to have 1 million Māori language speakers by 2040. In order to accommodate for these speakers, Aotearoa will need digital systems to understand te reo Māori, whether it’s a Māori-speaking Siri, automatic captioning, or other types of assistive technologies. Māori should champion these technologies. It is their language. If anyone is to profit from te reo Māori it should be Māori and Māori alone, especially considering the fact that non-Māori once sought to make the Māori language extinct. Our position is only Māori should be selling Māori language as a service.
But when technologies like Whisper are open sourced, it's not a matter of if but when will Big Tech and other "AI" companies start profiting from selling indigenous language tools and services back to our own communities. There are plenty of examples where this has already happened, and the issue of land is one in particular. Many indigenous peoples have had their land stolen from them and today, if they've managed to beat all odds against them to be in a position where they’re able to, they have to buy back the very land that was taken.
We also want to create opportunities for Māori, Pacific peoples, and other indigenous peoples to engage in high value STEM-related jobs in a sector dominated by White and Asian men. By empowering local indigenous organisations to build these tools, we're creating more opportunities for their local communities and people, rather than outsourcing those jobs to people who have no vested interest in the survival of languages.
Who Should Decide?
One of the biggest debates around these large models being released to the public is who should decide whether they should or shouldn't be open sourced. After first publicly releasing their GPT model, OpenAI backtracked "due to our concerns about malicious applications of the technology." They haven't, however, noticed any issues with publicly releasing Whisper. Have they done enough to ensure no harm can be had from Whisper, or are they making decisions similarly to Meta who've decided it's best for humanity if they give their technologies away for "free"? OpenAI, Meta, and other Big Tech have the hubris to decide on behalf of humanity.
Ultimately, it is up to Māori to decide whether Siri should speak Māori. It is up to Hawaiians to decide whether ʻōlelo Hawaiʻi should be on Duolingo. The communities from where the data was collected should decide whether their data should be used and for what. It's called self determination. It is not up to foreign governments or corporations to make key decisions that will affect our communities.
The communities from where the data was collected should decide whether their data should be used and for what.
But then it gets complicated. Who in those communities decides on behalf of the community? Does a single individual from that community speak on the community's behalf? No. Does a government department such as Te Taura Whiri i te Reo Māori speak on behalf of Māori to make key decisions around te reo Māori? No. We quickly get into the internal politics of indigenous peoples and their communities, and that's when you need to stay in your lane.
Our organisation seeks advice and support from our kaumātua (elders). They help guide us in making key decisions for our people; in fact, the kaumātua decided in 2013 that Te Hiku Media should build a digital platform and put the language of the people online so that those who live outside of the haukāinga (local tribal lands) could have access to their language and culture. In building te reo Māori tools, we've sought the advice and the support of kaumātua and of people who've worked in the language revitalisation and Māori sovereignty space for decades. We are reminded that we are a blip in time. Data sovereignty didn't start 8 years ago, as one AWS employee put it, it started the day of first contact with the coloniser. Indigenous people have been fighting for their rights for centuries. So many people have come before us. They marched to parliament. They wrote and legislated the Māori Language Act. Many of these people who fought so hard have since passed away. Today we continue that fight in a digital society ensuring the final frontier of colonisation, the extraction of our data, fails.
We've created climate change by disrespecting our environment. So our final message is a simple one: we must respect data. Respect it as indigenous people have respected their environments. Respect data so that we may prevent the catastrophic harm that comes from the pursuit of technology without responsibility, accountability, and thinking that technology is inevitable. Guns, germs, and steel did not lead to the inevitable destruction of our planet and its indigenous peoples. Imperialism, capitalism, and self-interest did.